When learning a language, different learners need to focus on different things. That is a statement on the scientific level of “No shit, Sherlock”! Yet, most language training does not adapt to the learner, the learner is almost expected to adapt to it. Not so for Linguineo! Every learner needs different support. And that is exactly what we provide with adaptivity! Adaptivity, you say? Yes, adaptivity! At Linguineo, we explore new ways to practice speaking and writing, seek out new technologies and boldly go where no language training program has gone before! We have been researching how adaptivity can be optimized for language acquisition. We even went above and beyond and developed fine-grained adaptivity. Which is even better than just plain old normal adaptivity. We don’t do normal. We can really nerd out on this stuff. A tailored approach for every individual learner, evidence-based and science-backed. It’s language learning, but not as you know it!
Adaptivity or not adaptivity? That is the conversational AI
So, what is adaptivity? Adaptivity is the process of adapting conversations and learning materials to the specific needs of a learner. But what does that mean?
Let’s consider Jim, who speaks a few languages. In Russian, he is a beginner, so he cannot yet use full sentences. With his intermediate level in Spanish, he is quite good at writing, but bad at speaking. His French is good, but his advanced grammar is bad. And his English is good, but his pronunciation is bad. So how can we help Jim?
Without adaptivity
Now, let’s see what happens if Jim starts his learning voyage without adaptivity. He starts a conversation. Imagine he gets the following interface in all the 4 languages he is practicing:
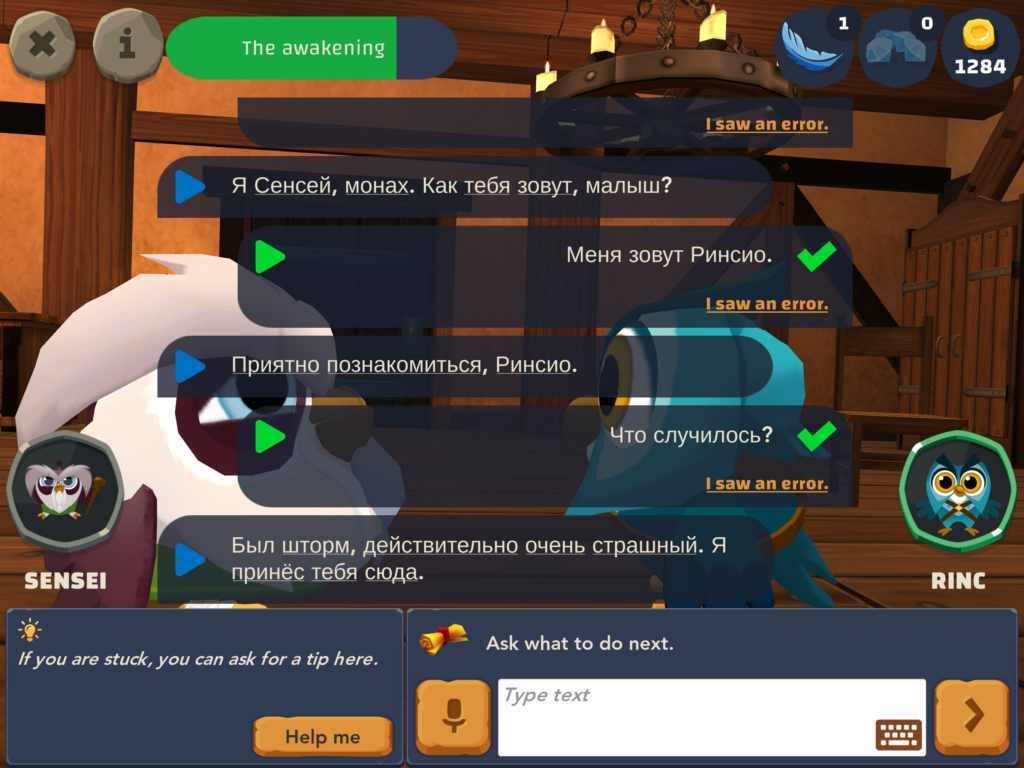
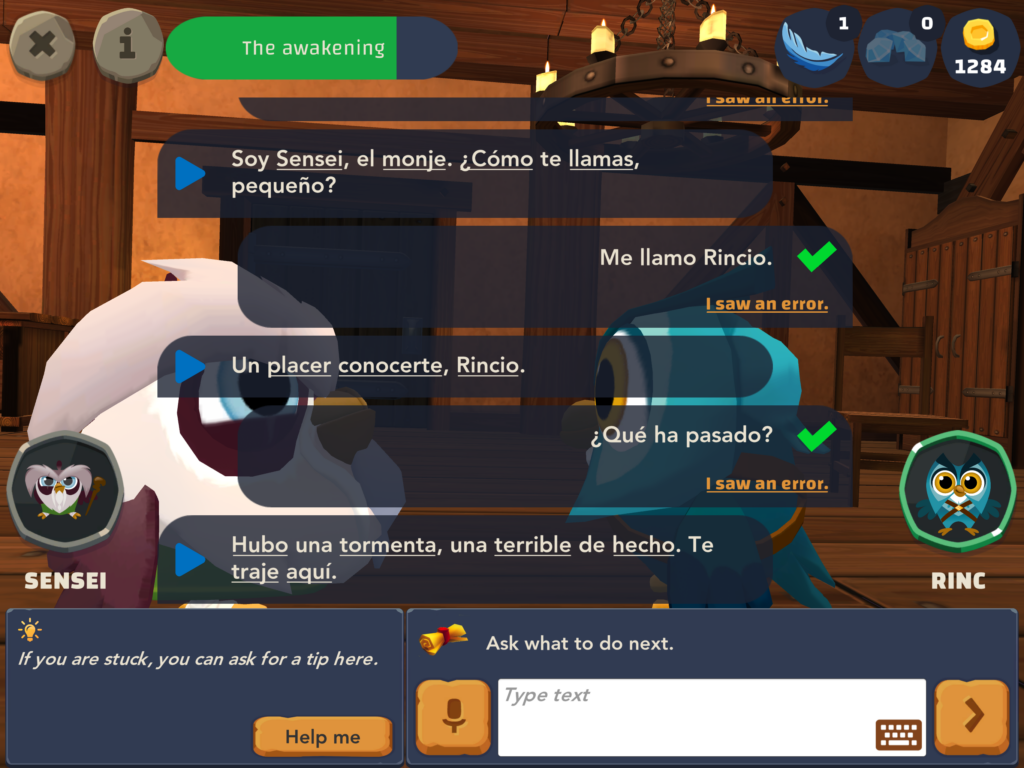
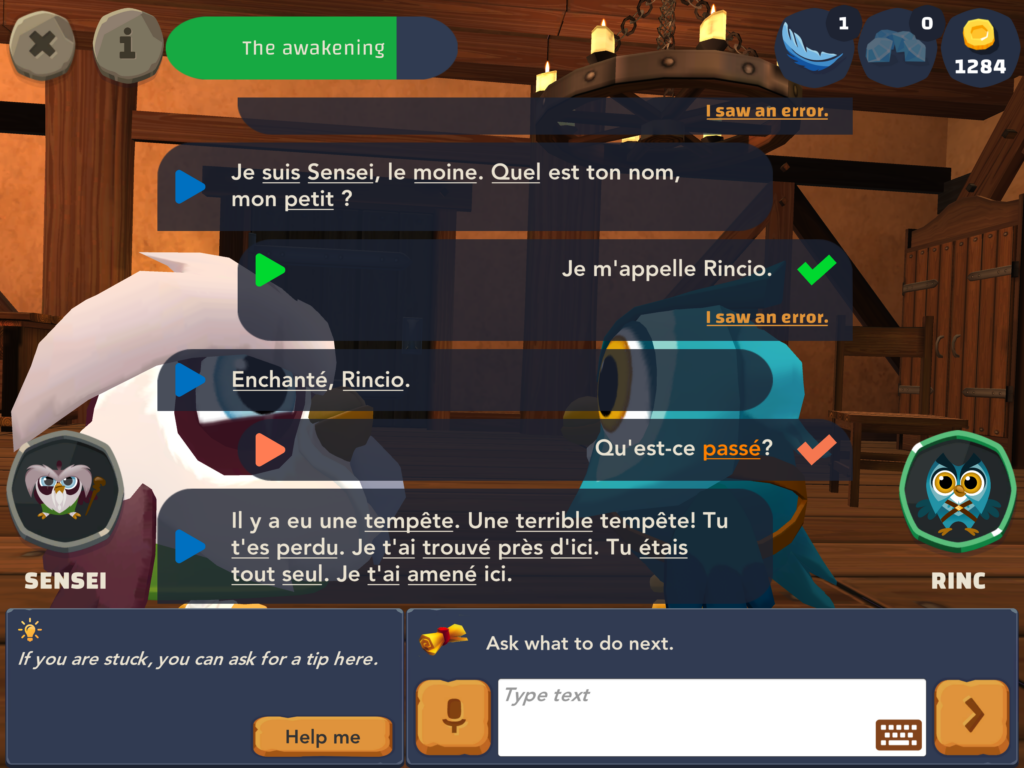
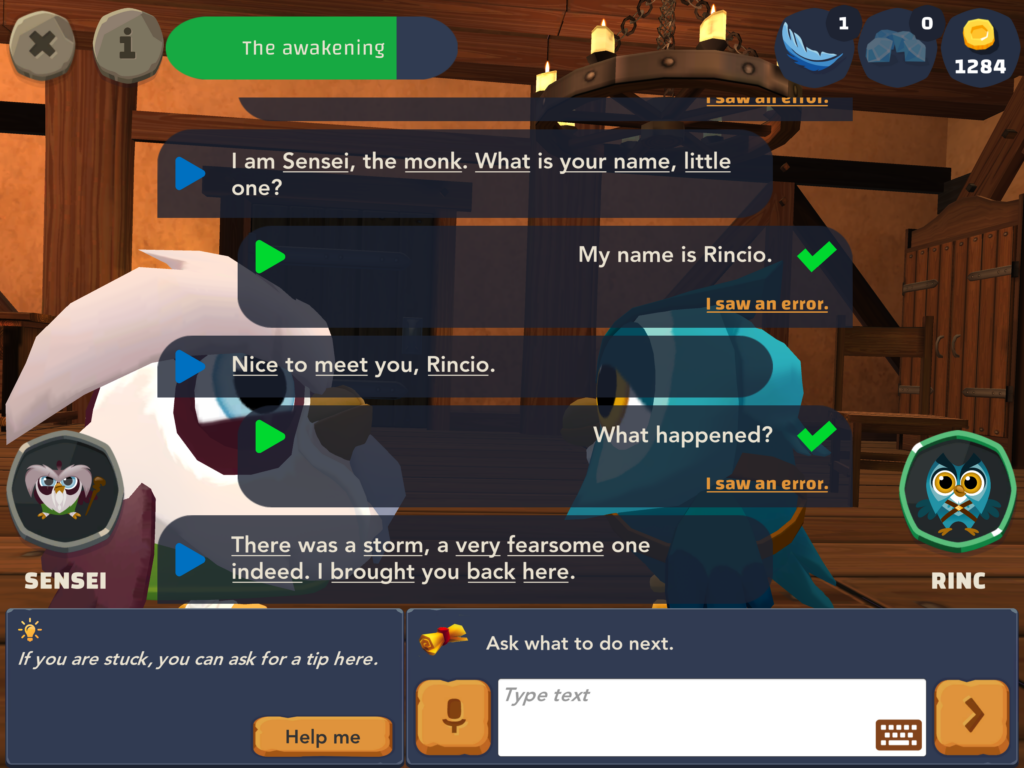
He starts practicing in Russian, but he has no idea what to answer. As a beginner, he needs support to form complete sentences. Да ладно, Шерлок. (No shit, Sherlock.)
But in Spanish he is good at writing, so he can write full sentences and decides to write. He knows that he should practice his Spanish speaking skills, but still decides to write.
In French he gets by using basic phrases, which are accepted by the bot. What he actually needs is using advanced grammar in French. Without adaptivity here, he is not improving his French skills where they need to be improved. Merde!
And when he starts practicing in English, he decides to speak instead of writing. The system understands his bad accent but doesn’t comment on it or gives feedback. This is due to the default setting, which will not comment on accent as it ‘understands’ what Jim says.
Depending on the language, the tool ranges from “plain unusable” (way too hard or way too easy) to “useful but it could be better”.
Jim is not receiving targeted feedback or tailored exercises. Targeted feedback or tailored exercises would help him improve much faster. The lack of this, leads to a lack of progress and increased frustration. So now let’s see what happens when we engage the adaptivity drive.
With adaptivity
With adaptivity, Jim receives a lot of support as a beginner in Russian. He gets to see tons of useful sentences with the translation in his native language. With adaptivity he gets to pronounce them:
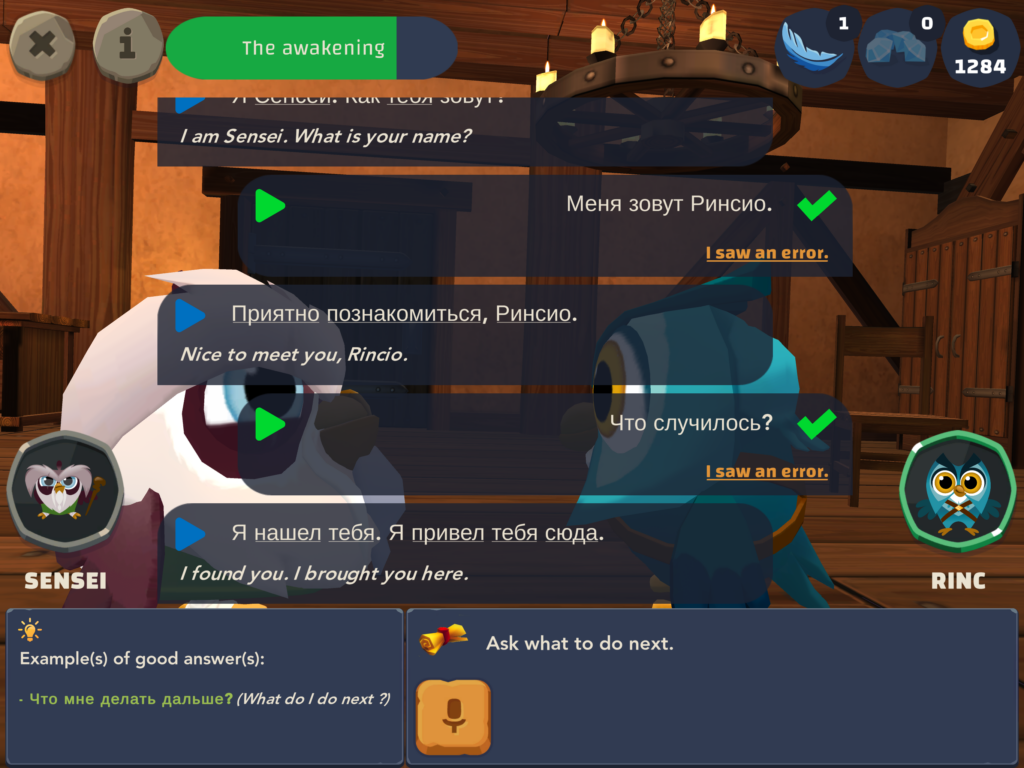
In Spanish, the system knows that Jim needs to improve his speaking skills. So, the system will force him to speak occasionally, if it knows he is able to do it. He can still switch, but the system will explain to him the need for speaking:
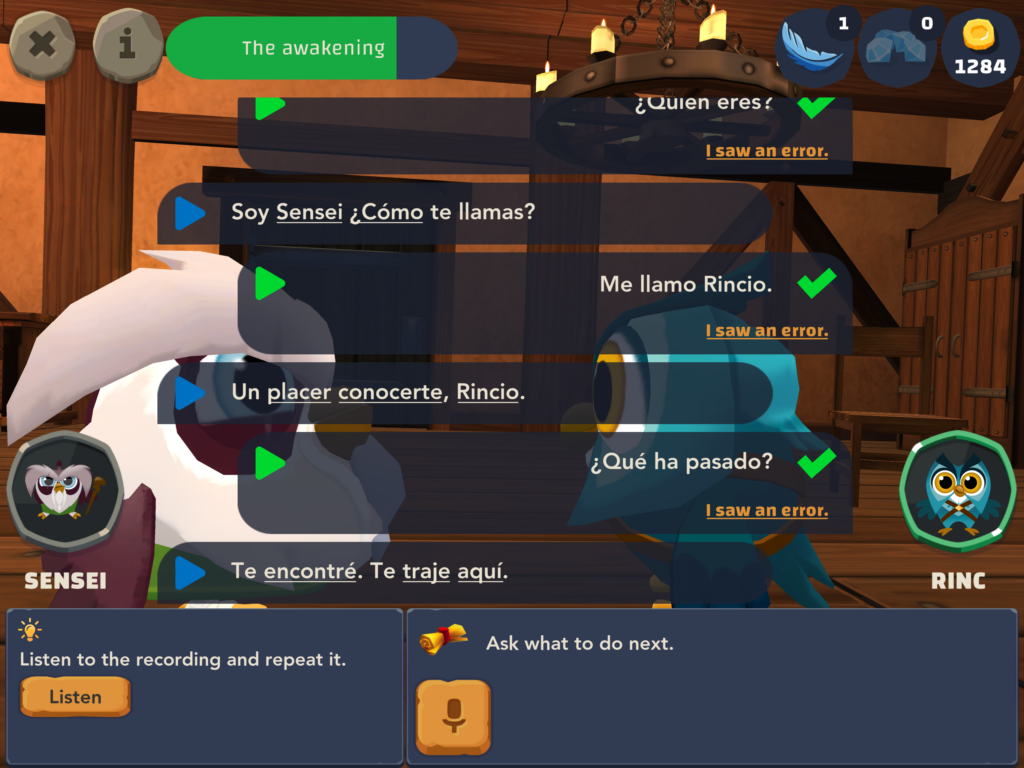
In French the system won’t let him make basic phrases. Instead, it will encourage him to use more advanced grammar. Dis-donc, ça, c’est quelque chose! The system will give him more corrective feedback in general to improve his grammar:
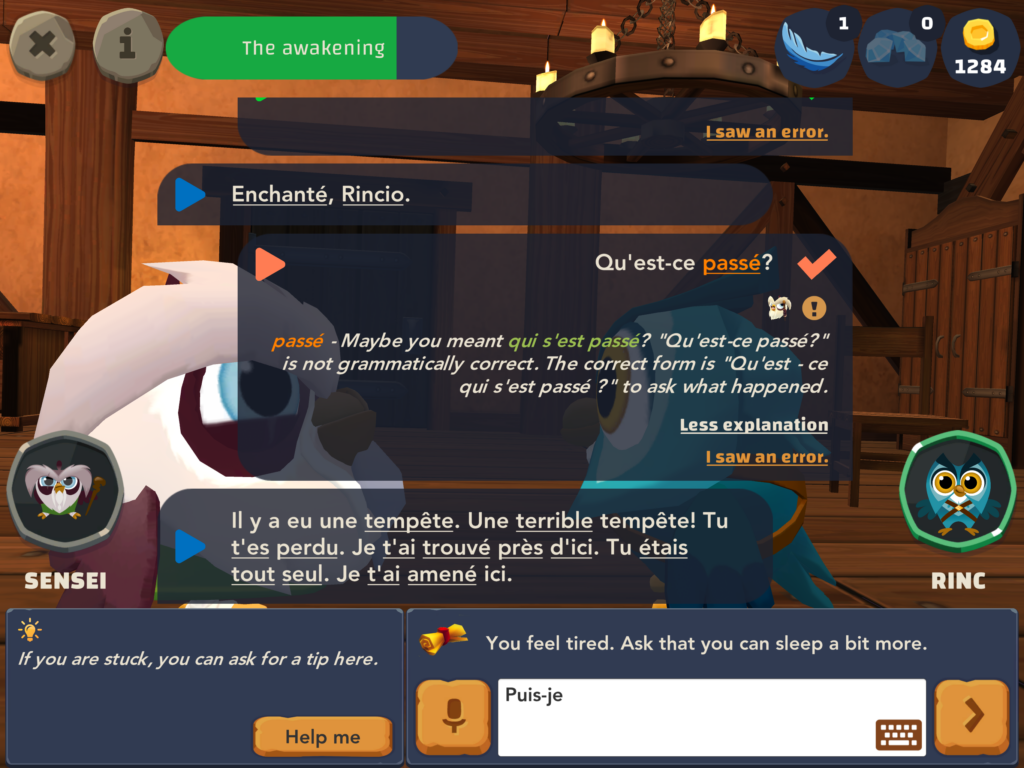
In English, pronunciation feedback is given on pretty much every utterance he does:
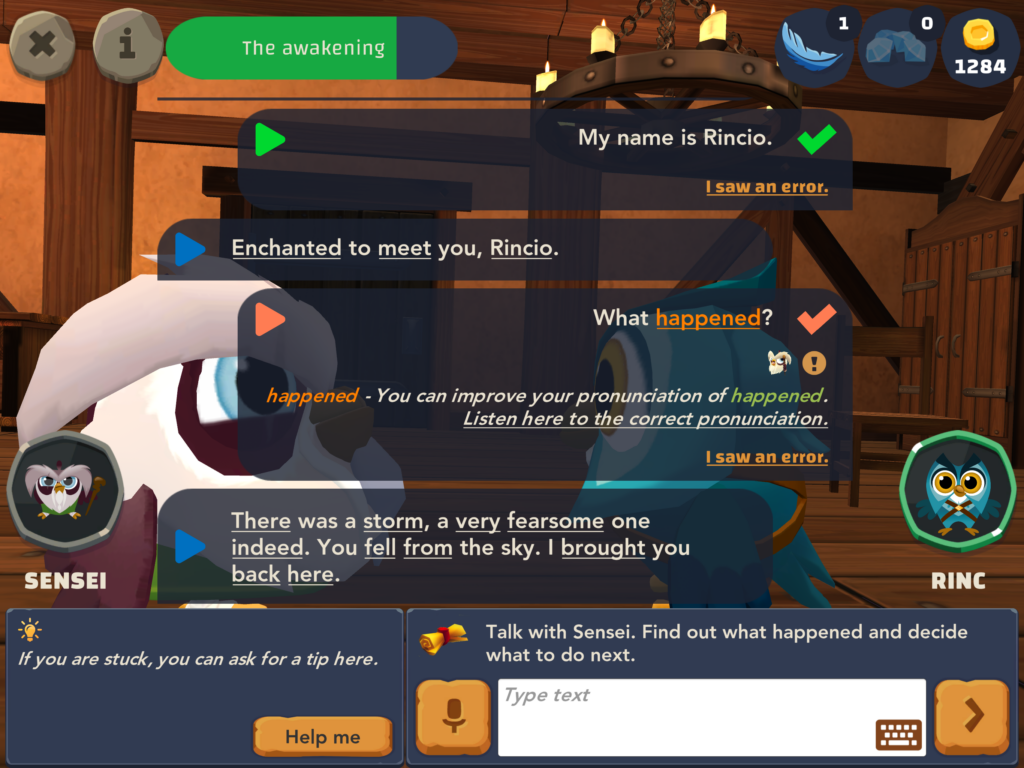
This personalized approach helps him make significant progress and stay motivated. Go Jim!
How could this be? Is it a kind of magic?
Now, you may be wondering, how does this work? Science, baby! To make it simple, we collect a rich dataset of the learner and item profile. This means that as the learner engages, we track their progress. By doing so, we can easily see in which areas learners may require more support or challenge.
For example, we already know Jim is good at writing in Spanish. The system knows that because Jim demonstrates that through his exercises. The system will slightly increase the difficulty of those exercises to identify areas needing more support and feedback. This way, we can ascertain that Jim needs more support for his Spanish speaking skills. Órale!
The system goes beyond this by analyzing learners’ performance on different items and then proceeds to identify patterns. For example, the system knows that Jim needs to improve his speaking skills and will force him to speak occasionally.
Using these insights, the system adjusts support tailored to suit the learner. This way the learner has an optimal learning experience.
Not satisfied with the simple explanation? Still on the scientific equivalent of “No shit, Sherlock” for you? Well good for you, wanting to know more! Keep reading!
All the science
Language learning apps typically include limited adaptivity based on learners’ language proficiency levels. Just like we showed in Jim’s case with the exercises he would get without adaptivity.
But with fine-grained adaptivity, we provide learning experiences tailored to the unique needs of each learner considering all language and personal aspects. Amazing, right? How do they do it!
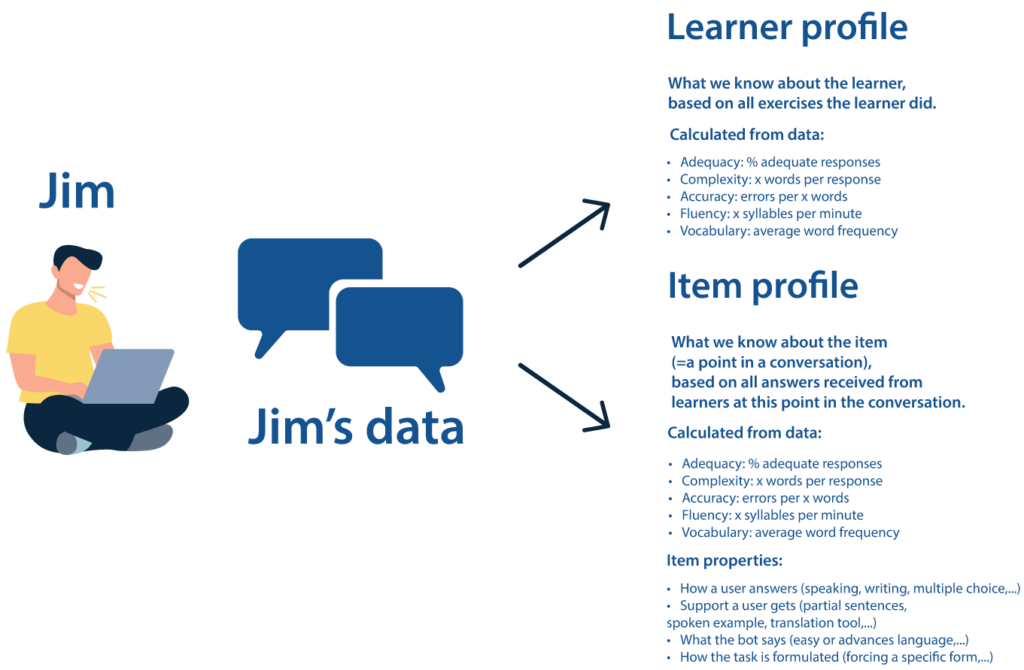
A first step towards adaptive complex tasks is to have an overall idea of a learner’s language proficiency. So, for adaptivity to work, we need to create a learner model. For this reason, we have developed a system consisting of two main components:
- A component that builds a detailed profile of learner and item:
We build extensive profiles of both learners and items. For learners, we analyze linguistic data such as utterances during tasks and calculate validated metrics that reflect their language proficiency. For example, we look at fluency, number of syllables per minute, accuracy, number of errors per words, but we also look at their behavior and metacognitive skills, such as how often they use the hints. By doing so, we create a holistic profile of a learner that explains the variation in their language performance and therefore can be used as basis for adaptivity.
2. A self-learning predictive component:
For an item we do the same, we try to estimate the difficulty of an item by looking at how learners score on average on that item. For example, we know the average accuracy, the average percentage of hints requested for that item, and so on.
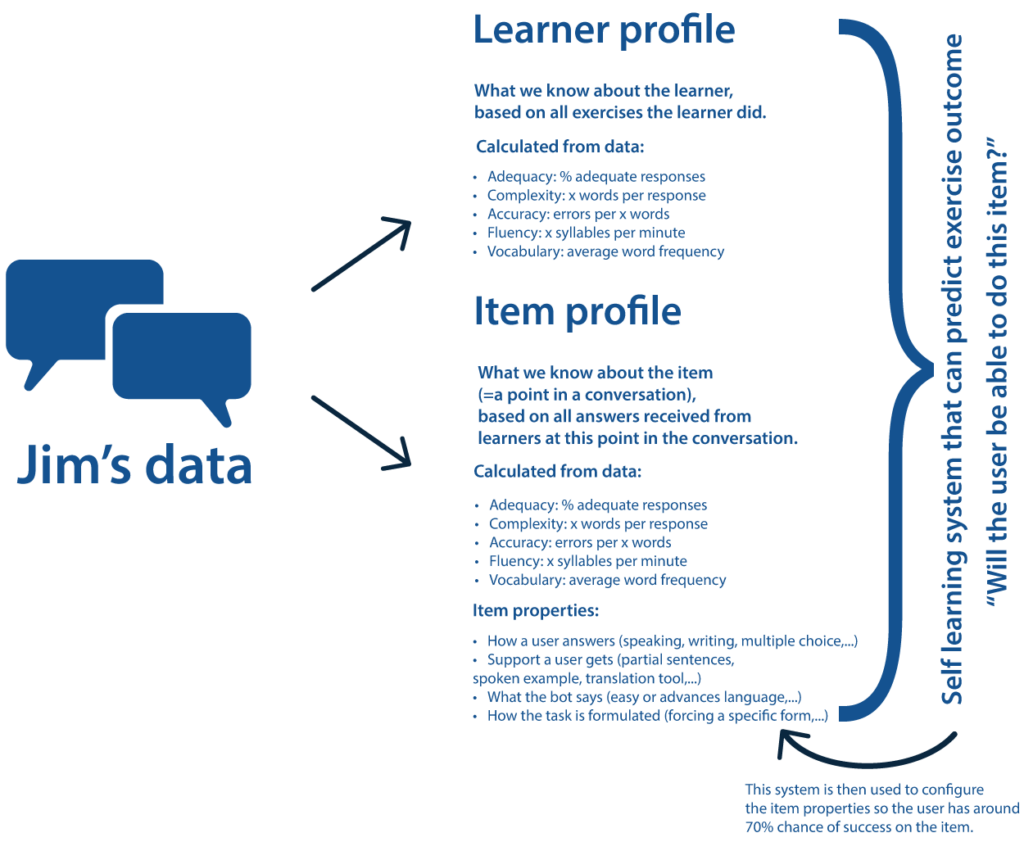
Based on learner and item profiles, predictions can be made about a learner’s performance. Learner and item profiles form a dataset to predict how a learner will perform on a task. Item profiles have fixed, and customizable parts related to support provided (e.g., writing mode, speaking mode, hints).
Adaptability involves determining the best item settings to ensure a 70% success rate for learners when encountering new tasks. And that self-learning system is going to determine the best form of support for that combination of learner and item.
To create this model, we conducted extensive research in collaboration with KU Leuven. The research showed that by leveraging our state-of-the-art language technologies and advanced analytics alongside a carefully selected dataset, we can construct a robust learner model that is capable of delivering fine-grained adaptivity in real-time. Moreover, the model is informed by theory in (second) language learning. This data-driven and theory-informed learner model is exactly what we need in order to enhance the effectiveness and efficiency of our language training solutions! Now that’s science! No, for real, it’s on the KU Leuven research portal and everything. Check it out here.
Fine-grained adaptivity is a powerful approach to personalize learning experiences and provide optimal support for each learner. At Linguineo, we use advanced technologies to create detailed profiles that can identify when something is too difficult or easy for a learner. This way, we ensure that everyone successfully masters a new language. Curious about adaptivity in language learning games? Try it here in Language Hero.